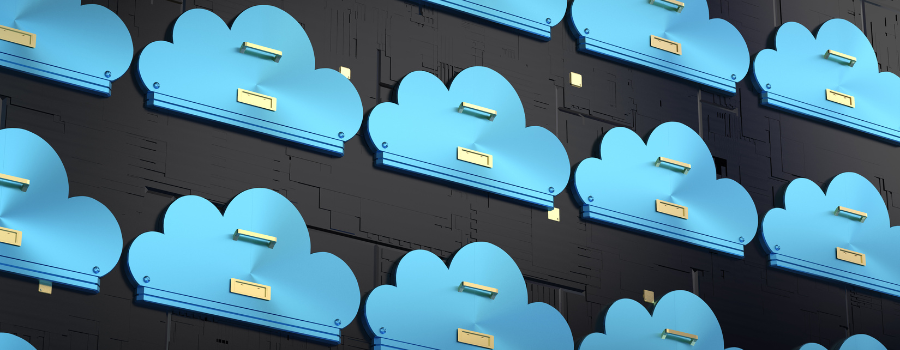
The Architecture, Engineering, and Construction (AEC) industry faces an unprecedented opportunity to harness the power of structured data for efficiency and innovation. At the heart of this potential is the data warehouse—a cornerstone for bringing information together from multiple sources into actionable insights and better decision making.
With rapid advancements in technology, the shift to cloud platforms, and the multiple stakeholders and systems used on a project, a well-designed data warehouse has vast potential for AEC firms striving to maximize and get control over all their data. Let’s explore the role of data warehouses in the industry, the challenges they address, and the steps to effectively implement them.
Why a Data Warehouse?
A data warehouse is a centralized repository that organizes data in a structured format. It is designed to collect, integrate, and store data from disparate sources, providing a foundation for analytics, search, streamlined reporting and to leverage AI.
The AEC Industry Data Challenge
- Fragmented Systems: AEC projects rely on multiple platforms and tools, each storing data differently. These systems often lack a unified structure, making it difficult to draw meaningful connections.
- Unused Data: A staggering 95% of project data is often unused, leaving critical insights untapped.
- Cost of Poor Data: Research indicates that 52% of rework stems from poor project data and miscommunication.
A data warehouse addresses these challenges by providing a unified structure that ensures data consistency, scalability, and interoperability across systems and stakeholders.
Building a Data Warehouse: Key Considerations
- Practical Steps to Begin
-
- Evaluate Tools: Choose platforms with strong data accessibility and modern APIs. Avoid tools that limit integration or rely on outdated interfaces.
- Define Objectives: Identify the key insights and reports you need from your data. Tailor your schema and integrate efforts to support these goals.
- Focus on Context: Ensure all data ties back to the context of individual projects for clarity and relevance.
- Invest in Scalability: Build with future growth in mind. A flexible architecture can adapt to new integrations and evolving project needs.
- Establish a Defined Schema
At the core of a successful data warehouse is a well-defined schema that dictates how data is organized and related. This ensures that:
- Relationships between data points are clear.
- The warehouse scales seamlessly as new data is added.
- Reporting and AI tools can efficiently extract insights.
- Integrating Disparate Systems
With multiple stakeholders and platforms in play, unifying data is challenging. While perfect alignment across systems may be impractical, focusing on essential data points can yield significant results. Tools with modern APIs (e.g., Microsoft, Autodesk, Procore) facilitate seamless data extraction and integration, enabling firms to construct a cohesive database.
- Adopt a Phased Approach
For firms grappling with legacy data, starting fresh with new projects ensures a clean, scalable foundation. Retrospectively integrating historical data can then be tackled gradually through structured mapping exercises.
The Connection between AI’s Potential and a Data Warehouse
With AI’s potential in analyzing large datasets, the importance of properly structured data for the AEC cannot be overstated. AI tools can only be as effective as the data they process and thrive on structured, high-quality data, which is exactly what a well-designed data warehouse provides. If the datasets fed into AI systems are inconsistent, incomplete, or poorly structured, the results can be misleading or even detrimental to the business.
A well-constructed data warehouse ensures that data is not only centralized but also formatted and validated for AI tools to process it effectively. Without this solid foundation, the power of AI is compromised, highlighting the critical need for a robust data warehouse that supports both current and future technology. In essence, the quality of your data infrastructure directly impacts the value and accuracy of the insights AI can deliver.
ProjectReady’s Data Warehouse
One example of a data warehouse for the AEC industry is our ProjectReady solution. By bringing project data together on the platform, clients receive a centralized, structured data warehouse that integrates information from various systems and stakeholders. This unified approach enables easy access to accurate insights, improves reporting, and supports AI-driven analysis, helping firms make better decisions and reduce rework. The data warehouse ensures that data is organized, scalable, and ready to drive innovation across projects.
The Path Forward
As the AEC industry embraces digital transformation, the need for a robust data warehouse is more evident than ever. Firms that prioritize data integration, leverage modern tools, and maintain a project-centric approach will position themselves to excel in an increasingly competitive landscape.
With the right strategy, a data warehouse becomes more than just a storage solution—it’s a critical enabler for innovation, efficiency, and future success.